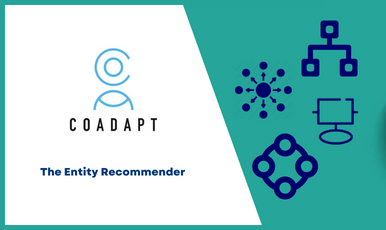
Everyday digital tasks can highly benefit from systems that recommend the right information to use at the right time. However, existing solutions typically support only specific applications and tasks. The Entity Recommender is a system that captures context across application boundaries and recommends information entities related to the current task. The user’s digital activity is continuously monitored by capturing all content on the computer screen using optical character recognition. This includes all applications and services being used and specific to individuals’ computer usage such as instant messaging, emailing, web browsing, and word processing. A linear model is then applied to detect the user’s task context to retrieve entities such as applications, documents, contact information, and several keywords determining the task. The system has been evaluated with real-world tasks, demonstrating that the recommendation had an impact on the tasks and led to high user satisfaction.
Figure 12: Screenshot from Entity Recommender
In the figure above a screenshot of the entity recommender, as an overlay window showing related documents and keywords.
1.1.1. Leadership and partners involved
The Entity Recommender work is led by the University of Helsinki as it is the larger contributor to the research. The research is further supported by AALTO.
Commercial recommendation systems that recommend similar objects then entities are found in online shopping and streaming portals. However, in both cases, these are not analyzing or aware of the context or tasks of the user, but mostly past history as well as collaborative filtering techniques. These systems have taken advantage of app-specific entities and data structures (such as movie recommenders), while other recommenders operate across apps by using generic textual input (like predictive keyboards) but they tend to recommend shallow textual structures.
Feature | Entity recommender | Content/commerce app/service | Operating system / Siri, Google |
Context-awareness | Task context. Learns the association of entities to the main tasks of the user | Only past ratings, and selections. | Limited context features (location, devices ), frequency. |
Entity model | Extensible entity model, across any application | Usually, only content or shopping items are recommended. | Entities can be documents, applications, and people. |
Interactive feedback | Online interactive feedback | Ratings are calculated over time. | No feedback. |
In the table above we summarise the most related and widely used recommenders. The closest implementation of recommenders is those in operating system assistants such as Google or Siri.
Currently, no system exists either in research or practice that is able to monitor the user learning relations of entities displayed on the screen across boundaries of applications, during the computer use and is able to recommend relevant entities during particular tasks. This functionality should be of interest to operating system developers/owners.
1.1.2 Exploitation roadmap
Step 1: Scientific dissemination and exploitation
The exploitation is mainly scientific and rests on high-level publications and publishing open-source code. Particular measures will be taken to disseminate the work and boost the download and uptake of the open-source code. In addition, a workshop at a major conference on entity recommendation will be planned involving research from both academia and industry.
Step 2: Learn from large Operating Systems owners.
This step includes setting up dialogue and collaboration with specific large companies that develop operating systems. This will include discussing how ageing users might require specific support. We will approach Apple Research. Here a principal scientist after a discussion has taken up some aspects of our research utilizing screenshots of apps to mine the user and its activity mostly for development purposes. We will initiate a dialogue to learn what is found to be interesting in the approach and possible obstacles and barriers. Similarly, we will utilize our contacts in Google and Microsoft research.
Step 3: Redesign, possible collaborative projects with industry.
Based on step two we will redefine an R&D agenda closer to the industry to maximize the impact.
Step 4: Investigate application to specific verticals and niche usage.
While our entity recommender works across any application and system, it can be easier to seek impact by applying it to a specific application area for example Law, Health, or other domains where the specific task support creates important efficiency improvements. However, this will require setting up first a research project to develop a demonstrator.