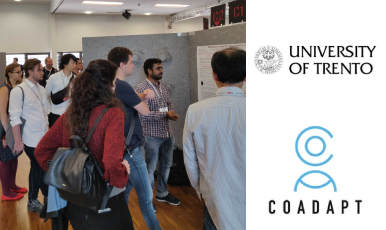
Please enjoy this explanatory article on the research coming from the 1st Use case of the Coadapt Application Study on creating a personalized Conversational Agent with comprehensive change support for ageing citizens based on data analytics focusing on high-level strategies and latest change psychology approaches considering wellbeing and digitalization across work and personal life. The target is to integrate in one system guided by a conversational agent, available knowledge on intervention programs and valuable knowledge comes from Personal narratives from the users.
The team at the University of Trento is studying how to create Machine Learning models for automatically understanding Personal Narratives (PNs), i.e., the recollection of events, thoughts, and facts from one’s own experience. Our work on PN understanding has been published so far first at the Annual Conference of the International Speech Communication Association (Interspeech) in 2019, the world’s largest conference on the science and technology of spoken language processing, and then at the International Conference on Language Resources and Evaluation (LREC) in 2020, a biennial major international conference in human language engineering.
What are Personal Narratives?
Personal Narratives (PN) are an important part of everyday conversations. We share stories with others and ourselves to make sense of our daily experiences. Through PNs we try to express and reflect upon our emotions. An example of PN could be, for example, telling a friend about a weekend trip with your family. The story may include mentions of the family members you met, activities you did with your siblings after a long time, the places you visited, and more importantly how all this made you feel happy. Another example of a PN could be telling about a negative event from your life such as a fight with your boss at work. In this case, you might talk about how your relations are with your boss, the reason for the fight, and how that affected other things. Recollecting or sharing such positive and negative memories affects our mood or emotional state. It might make us feel positive or negative, make us energized or passive. Why is it important to automatically understand Personal Narratives?
Personal Narratives are rich in information that could help better understand the mental state of the narrator. Creating models to automatically understand PNs could, therefore, be useful for several mental healthcare applications (e.g. early diagnosis of mental diseases, supervision of disease course, etc.). In particular, models able to automatically understand PNs could be crucial to extract information from users’ daily experiences useful to support therapists in their diagnosis.
“Modeling User Context for Valence Prediction from Narratives”
Aniruddha Tammewar, Alessandra Cervone, Eva-Maria Messner, Giuseppe Riccardi, Proceedings of the Annual Conference of the International Speech Communication Association (INTERSPEECH) 2019
Automated prediction of valence, one key feature of a person’s emotional state, from individuals’ Personal Narratives may provide crucial information for mental healthcare. In this work, we create Machine Learning models to predict the narrator’s state of mind, in terms of valence, from Personal Narratives. In particular, we investigate how the previously recounted narratives affect the narrator’s current state of mind. Our hypothesis is that context modeling might provide insights about emotion-triggering concepts (e.g. events, people, places) mentioned by narrators across multiple narratives that are linked to an individual’s state of mind.
We explore multiple machine learning techniques, (Support Vector Machines and an Attention-based sequence tagger based on Deep Neural Networks) to model narratives. We find that the models are able to capture inter-individual differences, leading to more accurate predictions of an individual’s emotional state, as compared to single narratives. Interestingly, our results indicate that emotion-triggering concepts beyond sentiment words (sad, happy) are useful to predict the emotional state of the narrator. These concepts included terms such as characters (e.g. grandfather, a friend), locations (e.g. swimming pool), and events (e.g. high school exam).
“Annotation of Emotion Carriers in Personal Narratives”
Aniruddha Tammewar, Alessandra Cervone, Eva-Maria Messner, Giuseppe Riccardi, Proceedings of the 12th Conference on Language Resources and Evaluation (LREC 2020)
In this work, we take the intuition arising from our previous work’s results and formalise it to propose Emotion Carriers, a novel concept for narrative understanding.
For Personal Narratives (PN), we define emotion carriers as the speech or text segments that best explain the emotional state of the narrator. Such segments may span from single to multiple words, containing for example verb or noun phrases. Advanced automatic understanding of PNs requires not only the prediction of the narrator’s emotional state but also to identify which events (e.g. the loss of a relative or the visit of grandpa) or people (e.g. the old group of high school mates) carry the emotion manifested during the personal recollection. In this work, we propose and evaluate an annotation model for identifying emotion carriers in spoken PNs.
Compared to other text genres such as news and microblogs, spoken PNs are particularly challenging because a narrative is usually unstructured, involving multiple sub-events and characters as well as thoughts and associated emotions perceived by the narrator. In this work, we experiment with annotating emotion carriers in speech transcriptions from the Ulm State-of-Mind in Speech (USoMS) corpus, a German PN dataset. We believe this resource could be used for experiments in the automatic extraction of emotion carriers from PN, a task that could provide further advancements in narrative understanding.